Learning-based Models in Medical Imaging: Closing the Gap towards Clinical Translation
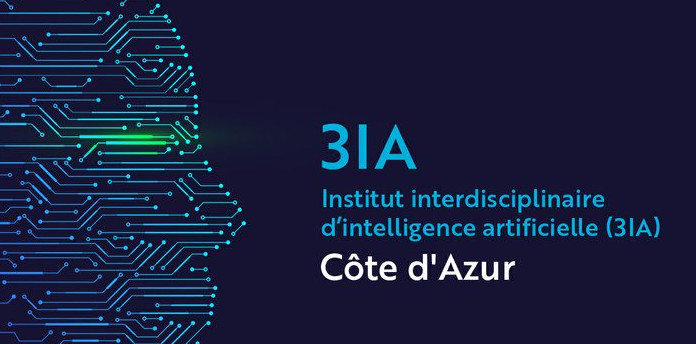
Learning-based Models in Medical Imaging: Closing the Gap towards Clinical Translation
Over the past decades, there has been a rapid progress in the machine learning (ML) field. Despite the numerously reported success stories of learning-based systems, its translation and wide use in critical domains faced with high-risk decisions, such as healthcare, remains an open problem. One of the remaining obstacles for successful translation is related to data being inherently complex, expensive to collect and to process. This leads to two specific problems: 1) high-quality data at training is often scarce and 2) small sample sizes and high dimensionality of the data are a source of bias, which leads to poor generalization. This project aims to develop novel reliable ML-based methodological frameworks that address the limiting factors associated to data, thus closing the current gap that stops effective translation of ML-based methods into clinical practice.
To this end, this project will define novel interactive ML-based algorithms that can learn gradually from user-provided weak annotations, as data becomes available, rather than demanding a fully annotated set from start. By relying on domain adaptation and generalization techniques, this research proposal will address poor generalization across domains/modalities of current ML-based methods. Within the project, there will be an important focus on the development of quality control (QC) techniques that continuously assess the performance of a (deployed) model. QC techniques will be conceived to generate alerts in the case of decreased performance of a system that indicates a degradation of its generalization capabilities. The activities of this chair application are structured around four axis. The first three focus on methodological and technical contributions. The final one will focuses on the clinical application of the developed methodologies. Specifically, it proposes the translation of the developed methods developed to concrete clinical use cases.