3IA Chair on Machine Learning for Computer Simulation
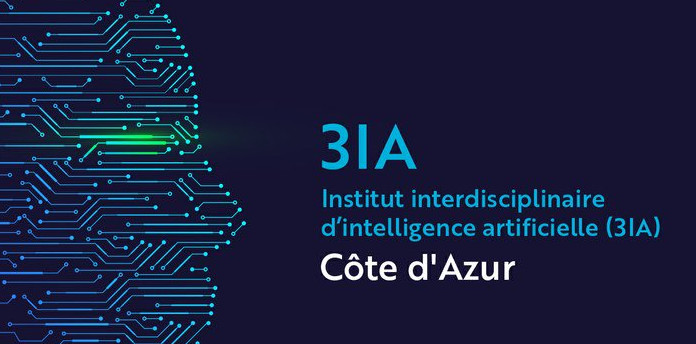
Machine Learning for Computer Simulation
Consequential decision-making requires a careful assessment of the resulting effects, before actually implementing that decision. For instance, for the decision of building a new facility in a city (e.g., dam, road, power plant), the policymaker must know the resulting effects on the environment beforehand. Similarly, to mitigate Climate Change, governments must assess how a candidate policy will affect the amount of CO2 emissions and the resulting future climate. Such assessments must be done before committing the decision of interest, because the decision may be costly to implement, or may be irreversible (i.e., once the decision has been implemented, we can never recover the state before the decision).
Computer simulation is a powerful tool that enables such assessments. It has been widely used in many scientific and engineering fields for modeling time-evolving complex real-world phenomena. Examples include simulators for climate change, natural disasters (e.g., flood, tsunami), epidemics (e.g., Covid-19), transportation (e.g., traffic), and industrial manufacturing processes, to name a few. However, a key question must be addressed before using simulations for decision-making: Can we trust the results of computer simulation? A simulator is just an approximation of real-world phenomena, and its reliability totally depends on its quality of approximation. Therefore, for reliably using computer simulation in decision-making, we first need to quantify and improve the quality of approximation. These are the validation and calibration tasks that I deal with in this project.
This project aims to develop generic techniques to improve the reliability of computer simulation, based on machine learning (or AI). This topic is crucial for our society, given the widespread use of computer simulation in high-impact decision-making (e.g., policies on climate change and Covid-19). However, the literature on simulation calibration and validation is far from mature, as has been discussed for climate simulation. Machine learning can play a key role here, and I hope to contribute to it as a 3IA chair.